GCP Cost Optimization: Strategies for Visibility and Control
Rabbit Team
7 min read
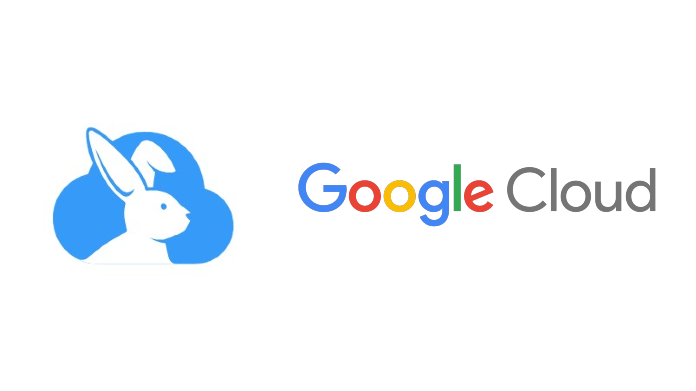
75% of companies overspend their cloud budgets. What starts as an exciting migration often ends with finance teams and engineers at odds over escalating Google Cloud Platform (GCP) expenses.
We’ve compiled strategies that have helped organizations like Bitrise, ResearchGate, and many other Rabbit customers transform their approach to Google Cloud spending—typically saving 30% and allowing engineering teams to focus on innovation instead of cost-cutting.
Behind these statistics lies a deeper challenge: the disconnect between cloud’s promise and its operational reality. Let’s examine the transparency gap that creates friction between teams and how bridging it transforms cloud cost management.
The Cloud Cost Challenge
Promise vs. Reality
“We migrated to GCP expecting to cut costs, but three years in, we’re spending more than ever - and I can’t even tell you exactly where the money’s going.”
For GCP users, the platform’s rich feature set creates a dilemma. The very tools that empower your team to build innovative solutions also introduce layers of pricing complexity that aren’t obvious until the bill arrives.
The Transparency Gap
When we started working with a mid-sized fintech company, they had been paying for hundreds of unused disks and idle virtual machines for nearly 18 months. Nobody noticed because the costs were buried in aggregate billing data.
This gap manifests in four critical ways:
- Service-level blindness: You know you’re spending $50,000 on Compute Engine, but which instances are driving costs?
- Allocation challenges: Without proper tagging, cost accountability becomes impossible.
- Technical complexity: Even seasoned engineers struggle with GCP’s pricing models.
- Delayed visibility: By the time most organizations detect a cost spike, it’s too late to prevent the overrun.
The Shift: Engineering Ownership
One engineering leader put it bluntly: “We only started caring about cloud costs when our CEO showed up at our stand-up with the monthly bill.”
What’s needed is a solution built specifically for Google Cloud that bridges the gap between engineering and finance by providing:
- Real-time visibility into costs across all GCP services
- Service-specific optimization recommendations
- Automation capabilities that eliminate tedious manual tasks
- Frameworks that align teams around cost goals without creating blame
Cost optimization works best when it’s not forced on engineering teams. When engineers can actually see where cloud money is going, they often find ways to cut waste without slowing down development.
It’s not about restricting innovation - it’s about giving teams the information they need to make smarter technical decisions that happen to cost less too.
Cloud Cost Management for Small to Medium Companies
For businesses with 50-400 employees spending between $20,000-$400,000 monthly on GCP without deep expertise, common pain points include:
- Inefficient resource management
- Unused Committed Use Discounts (CUDs)
- Idle resources
- Incorrect pricing models
- Engineers spending valuable time on cost optimization instead of building features
Rabbit’s Approach
Visibility and Analysis
- Service-level cost breakdown for BigQuery, Google Kubernetes Engine (GKE), and Compute Engine
- Resource-level insights down to individual instances, clusters, or datasets
- Business metrics integration to evaluate Return on Investment (ROI)
One customer discovered $5,000 in monthly savings on their first day simply by identifying idle Compute Engine instances.
Optimization Tools
- Right-sizing recommendations for over-provisioned resources
- Commitment planning guidance without unnecessary lock-in
- Resource cleanup for unused resources
Automation and Control
- BigQuery Autoscaler adjusts slots to reduce waste by up to 40%
- Real-time anomaly detection identifies cost spikes 70% faster than native monitoring
- Kubernetes agent tracks and applies real costs with a 15-second sampling rate
One client reduced their BigQuery compute spending from $100,000 to $66,000 monthly using Rabbit’s BigQuery Autoscaler, with zero manual intervention.
For organizations with significant data operations, BigQuery often represents one of the largest components of GCP spending. The platform’s analytics capabilities come with a pricing structure that requires specialized optimization strategies.
Let’s examine how data teams can tackle these unique challenges to reduce costs without sacrificing performance or insights.
Controlling Cloud Costs for Data Teams
BigQuery’s Complex Pricing Model
BigQuery’s pricing includes:
- Processing costs (pay for each query processed)
- Storage costs (prices decreasing for unused tables)
It offers two fundamentally different pricing models:
- On-demand pricing: Pay for bytes processed per query
- Slot-based pricing with reservations: Pay for dedicated query processing capacity
Real-World Impact
A marketing analytics firm discovered they had been running a costly report-generating query hourly for months. By adjusting the frequency to run just once per week, they reduced costs by 98.5%, saving over $5,000 monthly on a single query.
How Rabbit Helps Data Teams
Granular Cost Transparency for BigQuery
- Compute costs by individual queries
- Three-way view into Account, Query, and Tables data
Query Analysis and Optimization
- Groups similar queries to compare related executions
- Identifies suboptimal queries
- Offers partitioning and clustering recommendations
One customer restructured a mission-critical data pipeline, reducing processing costs by 42% without changing output or performance.
BigQuery Storage Cost Optimization
- Storage model recommendations based on data characteristics
- Unused table identification
- Retention setting monitoring
A media company discovered thousands of old tables, freeing up over 100TB of storage and saving approximately $5,000 monthly.
BigQuery Autoscaler
- Adjusts max slots on a second-by-second basis versus GCP autoscalers’ 60-second minimums
- A marketing technology customer reduced wasted slots from 48% to 28%, saving close to $40,000/month
Practical Recommendations
- Set up different reservations for different workload types (production, staging, development)
- Consider on-demand pricing for queries with small data reads but complex processing
- Implement appropriate retention policies and cleanup strategies for all datasets
These BigQuery optimization strategies work well for established GCP users, but organizations during migration face different challenges.
Moving workloads to GCP introduces unique cost considerations that require careful planning. As one customer discovered, “Our first month after migrating to GCP, our bill was triple what we expected.”
Navigating Cloud Costs During GCP Migration
The Migration Cost Challenge
“Our first month after migrating to GCP, our bill was triple what we expected.”
Migration presents unique challenges from two common approaches:
- Lift and shift - Faster but often not optimized for cost or performance
- Application modernization - Better optimized but requires more upfront work
Rabbit’s Approach
Forecasting and Budget Planning
- Forecast recurring monthly expenses expected post-migration
- Provide cost transparency to decision-makers before fully committing
A healthcare organization built a detailed cost model showing both immediate post-migration costs and optimized long-term expenditures, securing executive buy-in.
On-Prem to Cloud Cost Comparison
- Tools to understand Total Cost of Ownership (TCO) differences
- Visibility into how cloud costs compare to on-premises expenses
Phase-Based Migration Tracking
- Track cost progression throughout migration phases
- Identify when a phase completes and optimization can begin
A financial services company optimized each phase as it completed rather than waiting for the entire migration to finish.
Migration-Specific Optimization
- Identify over-provisioned resources from initial migration
- Flag lift and shift workloads that could benefit from modernization
A retail customer analyzed freshly migrated GKE environments, discovering they could reduce costs by 42% by adjusting resource allocations and implementing spot instances for development environments.
Best Practices
- Establish cost baselines early
- Implement continuous cost monitoring
- Optimize incrementally after workloads stabilize
- Integrate cost awareness into migration planning
Companies that integrate cost awareness throughout their migration process typically achieve 25-40% lower overall migration costs.
While migration presents its own cost challenges, enterprise organizations face these issues at significantly larger scale and complexity.
As cloud environments grow, siloed teams, complex data pipelines, and sophisticated chargeback requirements create additional layers of cost management challenges that require more comprehensive solutions.
Conclusion
Cloud cost management starts with visibility, continues with smart optimizations, and becomes most effective when teams have the right context to act. For smaller and mid-sized companies, solving inefficiencies and automating routine tasks can already lead to significant savings.
But these challenges grow with scale. In the next part of this series, we’ll focus on what cost optimization looks like in complex enterprise environments—covering cross-team transparency, chargeback models, and advanced optimization strategies for large-scale GCP use.